Have you ever pondered over the intricate dance of efficiency that underpins the seamless delivery of goods worldwide? In the labyrinthine realm of logistics, where precision and speed are paramount, the integration of advanced supply chain data analytics emerges as a beacon of innovation and reliability.
Imagine a logistics landscape where every decision, from route planning to inventory management, is guided not just by intuition but by a symphony of data-driven insights. This convergence of technology and strategy isn’t just a theoretical concept but a transformative reality reshaping supply chains globally.
Join us as we explore how harnessing the power of data analytics not only optimizes operations but also enhances decision-making, reduces costs, and fortifies resilience in an increasingly interconnected world.
Benefits of Advanced Data Analytics in Logistics
Advanced data analytics holds the potential to transform logistics operations across various dimensions, driving significant improvements in operational efficiency, decision-making, cost management, and risk mitigation.
- Improved Operational Efficiency: One of the most compelling benefits of advanced data analytics in logistics is its ability to streamline processes and enhance overall efficiency. By analyzing historical and real-time data, logistics managers can identify inefficiencies, optimize routes, and streamline workflows, leading to faster delivery times and reduced operational costs.
- Enhanced Decision-Making: In the supply chain analytics consulting world of logistics, decisions need to be made swiftly and accurately. Advanced data analytics empowers decision-makers by providing actionable insights derived from vast datasets. Whether it’s adjusting inventory levels based on demand forecasts or rerouting shipments in response to real-time traffic conditions, data-driven decision-making minimizes risks and maximizes operational effectiveness.
- Cost Reduction and Savings: Controlling costs is paramount in logistics. Supply chain analytics services enable companies to identify cost-saving opportunities across the supply chain. By optimizing routes, reducing fuel consumption, and minimizing inventory holding costs through better demand forecasting, organizations can achieve substantial savings while maintaining service levels.
- Better Risk Management: Logistics operations are inherently exposed to various risks, such as supplier delays, natural disasters, or geopolitical events. Advanced data analytics enhances risk management by providing predictive insights into potential disruptions. By anticipating and preparing for these risks, logistics managers can implement proactive measures to mitigate their impact and ensure continuity of operations.
Key Applications of Data Analytics in Logistics
The application of data analytics in logistics spans a wide array of critical functions, each contributing to operational excellence and customer satisfaction.
- Route Optimization: Efficient route optimization is essential for minimizing fuel costs and reducing delivery times. Advanced data analytics algorithms analyze factors like traffic patterns, weather conditions, and delivery constraints to recommend the most efficient routes in real-time.
- Demand Forecasting: Accurate demand forecasting is crucial for maintaining optimal inventory levels and meeting customer expectations. By analyzing historical sales data, market trends, and external factors, data analytics enables logistics planners to predict demand with greater accuracy, thereby reducing stockouts and overstock situations.
- Inventory Management: Balancing inventory levels is a delicate task that directly impacts costs and service levels. Data analytics models optimize inventory by considering demand variability, lead times, and cost constraints, ensuring that warehouses stock the right products in the right quantities at the right time.
- Fleet Management: Effective fleet supply chain management analytics hinges on optimizing vehicle utilization, maintenance schedules, and driver efficiency. Advanced data analytics tools monitor vehicle performance metrics in real-time, enabling proactive maintenance and route adjustments to minimize downtime and maximize fleet productivity.
- Real-Time Tracking and Visibility: Real-time tracking and visibility into shipments provide stakeholders with actionable insights into delivery status, location, and potential delays. By leveraging IoT sensors and supply chain analytics platform, logistics managers can track goods throughout the supply chain, improving transparency and customer satisfaction.
- Supplier Performance Analysis: Evaluating supplier performance is critical for maintaining a reliable and cost-effective supply chain. Data analytics tools assess supplier lead times, quality metrics, and pricing trends, enabling informed decisions regarding supplier selection and relationship management.
- Predictive Maintenance: Unplanned equipment downtime can disrupt logistics operations and incur substantial costs. Predictive maintenance powered by data analytics uses machine learning algorithms to forecast equipment failures based on usage patterns and sensor data, allowing maintenance teams to preemptively address issues before they escalate.
Implementing Data Analytics in Logistics Operations
While the benefits and applications of advanced data analytics in logistics are clear, successful implementation requires careful planning and execution.
- Developing a Data Analytics Strategy: A robust data analytics strategy begins with defining clear objectives aligned with business goals. Whether the focus is on improving delivery times, reducing costs, or enhancing customer service, stakeholders must outline key performance indicators (KPIs) that will guide analytics efforts.
- Selecting the Right Analytics Tools and Software: Choosing the right supply chain data analytics software is crucial for deriving actionable insights from data. Whether opting for integrated supply chain management platforms or specialized supply chain predictive analytics tools, the choice must align precisely with operational requirements and scalability goals. Each decision impacts how efficiently logistics operations can harness data to optimize processes, reduce costs, and enhance overall performance in a competitive market landscape.
- Integrating Data from Multiple Sources: Logistics data originates from diverse sources such as ERP systems, IoT devices, and external databases. Integrating data seamlessly across these sources ensures a holistic view of operations, enabling comprehensive analysis and informed decision-making.
- Building a Skilled Analytics Team: Effective implementation of data analytics relies heavily on a skilled team capable of interpreting data insights and translating them into actionable strategies. Hiring or training personnel proficient in data science, statistics, and logistics is essential to maximize the value derived from supply chain data analytics initiatives. Their expertise ensures that data-driven decisions lead to improved operational efficiency, cost savings, and strategic advantages in a competitive market environment.
In conclusion, the integration of advanced data analytics into logistics operations represents a transformative opportunity for businesses seeking to gain a competitive edge in today’s global marketplace. By harnessing the power of data analytics for supply chain visibility and analytics, organizations can optimize processes, reduce costs, mitigate risks, and deliver superior customer experiences.
As technology continues to evolve, leveraging supply chain analytics companies and platforms will be essential for staying ahead of the curve and driving continuous improvement in logistics efficiency and effectiveness.
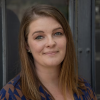
Lynn Martelli is an editor at Readability. She received her MFA in Creative Writing from Antioch University and has worked as an editor for over 10 years. Lynn has edited a wide variety of books, including fiction, non-fiction, memoirs, and more. In her free time, Lynn enjoys reading, writing, and spending time with her family and friends.